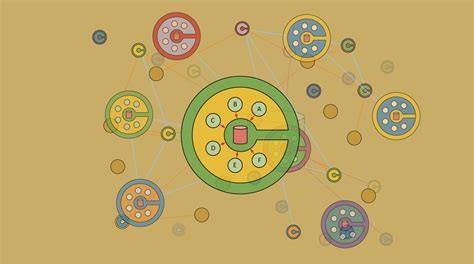
Data management and work in nonprofit and social service agencies is generally less developed than the big data storage and analysis systems of platform capitalism. Data work in nonprofits is increasingly central to their operation, however, and the sector is experiencing rapid adoption of corporate data platforms. Given the value commitments of the sector, and this unique moment of development, the sector also presents strong opportunities for cooperativization. This blog essay explores the context of the sector and the opportunities for different approaches to data work, then argues for the value of exploring data cooperatives as a potential solution.
Data work in nonprofits and social services represents a core tension in the sector. The beginnings of social service work in the United States and UK involved surveilling clients to differentiate between the “deserving” and “undeserving”, which evolved into tracking clients to understand progress toward (externally imposed) goals and to improve services, a practice originally heralded as “scientific charity.” In the last 30 years, policies like the US Government Performance and Results Act (1992) spurred long-lasting “accountability” movements, where funders demand specific and measurable outcomes and social service agencies must respond by developing data systems to meet these demands.
Social service agencies often have few funds to invest in systems and infrastructure development, especially for expensive database technologies. Thus, they rely on homebrew and ad hoc systems, wherein they develop diverse and usually incompatible systems using a hodgepodge of available technologies, including file folders, Excel spreadsheets, Google Forms, and the like. However, developments in the availability and affordability of technologies, and the push to consolidate and centralize social service provision through movements like Collective Impact, are leading to the rapidly increasing use of platform technologies that link and centralize client monitoring across many services.
Collective Impact strategies draw together nonprofit and government organizations in structured ways in efforts to “move the needle” on particular social issues, using shared finances, activities, and data tracking. This includes developing shared metrics and measurements, as well as data platforms, to collect, track, and manage data. Collective Impact and the growth of several for-profit data systems for nonprofits, helped push the social service sector toward corporate data platforms. This led to a trend toward platforms with centralized ownership, designed by a narrow group of stakeholders, and typically with little involvement by organizational staff, clients, and even leadership. These organizations dictate what data should be collected, which measurements are valid and valued, and what outcomes should be prioritized. This means local, community-based and operated organizations give up power to external entities to define program outcomes. These superordinate entities develop and manage data systems, and enforce participation by controlling funding. Decision-making is then more tightly driven towards the interests of those controlling the data systems.
While in theory these systems lead to increased data sharing and the possible improvement of service delivery, the actual impacts of these systems are understudied and unclear. My research, conducted with youth-serving nonprofits (a subset of social service agencies), shows that there are significant tensions between these external data demands, clients, and the staff who work with clients. These tensions are driven by corporate/funder demands on one side, pitted against staff and client concerns on the other. The latter group, in my research, understood demands for data to be part of ongoing power dynamics between those who “have” and those who do not. They understood their work to be advocating for and building toward justice alongside their clients, and believed data demands were often in opposition to this work. Finally, they were very interested in the many potential benefits they believed data could provide for their work – but believed that data systems needed to be built from the ground up by staff and their clients, rather than imposed from above.
I contend that nonprofits present excellent opportunities to grow platform cooperatives. While in general, platform capitalism is well-established and cooperatives in this context work against significant capital and resource inertia, the nonprofit sector is highly uneven in its current participation in capital-driven data systems. Many in the sector see the benefits of having data systems, but resent existing choices as profoundly dissatisfying and even demoralizing for staff and their clients. Nonprofits find themselves forced to buy into the few existing models available because they lack the resources or infrastructure to develop their own. Further, they work at the behest of funders’ demands for data, often experiencing data drift, in which their mission-driven work is dragged astray by corporate data systems that focus data collection on narrow interests.
Data cooperatives are a specific type of platform cooperative focused on collecting, linking, sharing, analyzing, and selling access to data. They are a type of data intermediary organization (which includes data commons, trusts, and unions) that springs from cooperative structures more generally. Data cooperatives represent a user- or worker- owned alternative to the surveillance and data hoarding of platform capitalism (which exerts significant control in the global economy through the use and sale of user data). As such, the data cooperative as a structure offers a decentralized form of data management that promotes collective economic power and owner-autonomy. This includes democratic member participation and governance, which involves shared decision-making about the sharing and use of data, as well as strategic directions for the cooperative. Governance may take place by direct or representative democracy.
There are currently a limited number of data cooperatives due to several barriers to establishing these entities, though there are many more data intermediaries that share similar governance and ethical commitments to cooperative structures (data commons, for example). Data cooperatives currently exist in industries like health and fitness, farming, location and mapping, shopping, citizen science, and even construction management, with varied success and longevity. Most are producer (member)-owned models. Reasons for becoming a member of a data cooperative include data altruism (sharing data to help others, often in health research) and data sovereignty (to retain power and control over one’s own data).
Nonprofits and social service agencies could organize their data systems as data cooperatives, banding together to share technology and infrastructure, while using collective governance to develop shared commitments and metrics. The benefits of data cooperatives in nonprofits and social services are numerous. They have the opportunity to:
- Improve referrals and communication between organizations for services in similar geographic regions (for example, shelter, food, transit, and mental health systems working together).
- Improve relevant services to clients by assessing overall client needs across service areas and linking available resources (such that any node in the cooperative can interconnect a client with any other useful nodes).
- Save on resources to implement and maintain data systems. While nonprofits cannot develop bespoke systems on their own, together investments in shared data infrastructures are possible. These could even become part of a business model for some cooperatives, inviting others to join the cooperative to gain access to data systems.
- Share data analysis. At present, nonprofits compete for grants and contracts by attempting to show that they are or will more effectively use resources toward particular funder goals. With shared analysis strategies, nonprofit data cooperatives could build collective rather than competitive analyses of their outcomes, demonstrating to funders the value of investing in particular areas.
- If many nonprofits band together, they gain negotiating power with funders over what data to collect, what matters, take back power to shape the narrative and discourse and move away from narrow accountability models. Many funders look for particular metrics because they are trendy or are implemented by existing corporate data systems, not because they are deeply committed to them. If an alternate model is commonly presented, many funders will be willing to adapt. Thus, nonprofits and clients would gain a seat at the table to negotiate priorities and measurements.
- Collective governance and routes to building further cooperation – while Collective Impact strategies theoretically encourage cooperation, shared vision and goals are usually established by a superordinate organization. A data cooperative could encourage other forms of cooperation, including the development of a ground-up shared vision. It would create an established vehicle and practice of collaborative governance that could extend further.
- Keep work and skill development local to the community. Many nonprofits are invested in growing local communities through employment and development. A data cooperative model would bring jobs in evaluation, data systems management and development, and the like back into communities and away from corporate investors.
While many nonprofits and their staff are dissatisfied with existing data systems, data systems are secondary to their greater social purposes and missions, and there are many barriers to establishing data cooperatives. Most nonprofits lack dedicated staff working on data related issues. It is much easier to outsource to existing corporate solutions than to work cooperatively toward others. Nonprofits typically have few resources to spare for infrastructural projects, leading to the development of homebrew systems, where staff create their own ad hoc data systems that solve current data management problems, but do not integrate easily with other systems and can be difficult to sustain. Nonprofit funders often want specific data, but this data varies by funder and changes over time, leading nonprofits to create frankenstein data systems that serve past and present purposes, but are inefficient, collect more data than necessary, and lead to burnout related to data collection for clients and staff. There are also few data analysis resources available for most nonprofits, meaning that staff spend precious time gathering data from clients, but never see results or benefit from this data. Data cooperatives would thus face many existing challenges related to data in nonprofit organizations.
For these reasons, and to my knowledge, few (if any) cooperatives for nonprofits and social services currently exist, although many corollaries do (for example, attempts to involve people in the civic life of their communities). Cooperative pools of data exist, specifically for donor management and fundraising, but these are owned and governed by corporations. Collective Impact strategies demonstrate some of the possibilities of these cooperatives, but they lack the orientation and commitment toward the ownership of those most directly involved. Building data cooperatives would solve a number of issues related to data ecosystems and the political economy of nonprofits, but also has the potential to build further cooperative work.
One example of a partial data cooperative for nonprofit agencies is the Chesapeake Monitoring Cooperative (CMC), a citizen science water monitoring organization constituted by community science initiatives around the Chesapeake Bay Watershed. Member organizations contribute data to the cooperative, which provides the infrastructure to integrate, store, and make accessible the combined data of members. The CMC provides resources for new member startup, supporting new contributing members through study design, methods evaluation, water quality training, and data interpretation. This model represents part of a data cooperative for nonprofits in that the cooperative is governed by leading organizations, with members contributing to a data pool, but not transparently participating in democratic governance of the organization.
There are several promising starting places for developing full data cooperatives. Starting nonprofit data cooperatives might first involve organizing with nonprofits (a bottom-up approach). While the social mission of nonprofits would align well with a data cooperative model, nonprofits would need to see demonstrable benefits and a route toward efficient and effective adoption within their organization. A data cooperative would need to provide clear and immediate tangible benefits for speaking to funders about program outcomes. It would need to be easy to integrate with existing organizational systems. Any technical infrastructure provided should have the input of frontline service workers, who would most regularly be using the system.
A bottom-up approach like this may be possible, and there are already models to work from. For example, in some areas, nonprofit IT systems are already organized as cooperative entities, contracting with nonprofits to provide shared services. These entities, often a nonprofit themselves, might be a starting place for housing a data cooperative. There are also models of open cooperation, including the OpenReferral protocol, which demonstrates an open standard for sharing and linking referral data between nonprofits.
Another route to developing a data cooperative could involve one or more funders organizing to develop cooperatives because they are a model that better fits their social commitments and would better serve nonprofit agencies. In this case, a funder might sponsor the creation of a data cooperative, inviting nonprofits to become members and passing management and governance to members over time. A combination of these two methods is most likely – organizing nonprofits and then convincing a funder to invest in the development of infrastructure.
Without full existing models, research on nonprofit data cooperatives is difficult. However, a possible next step is the assessment of needs, which also offers opportunities to start discussing and imagining possibilities with nonprofits. This is a next step in my own research, as I work with nonprofits to understand whether the landscape is fertile for data cooperatives in this sector, and what it might take to convene and grow them. Nonprofits and those interested in organizing with them can benefit from conversations with others about their collective data needs, and the exploration of cooperative models. Thoughtful funders could incentivize and support the creation of cooperative data systems for nonprofits, recognizing the value of developing shared, cooperative governance models. There is an opportunity, as the sector negotiates the tensions between values-driven work and the ease of corporate data platforms, for nonprofits and social service agencies to develop strong and sustainable data cooperatives. If they can do so, they might develop the power to gain more seats at the table with funders and decision makers to shape better futures with their clients.
Add new comment